Different types of hypothesis test
From Python for R users, p151
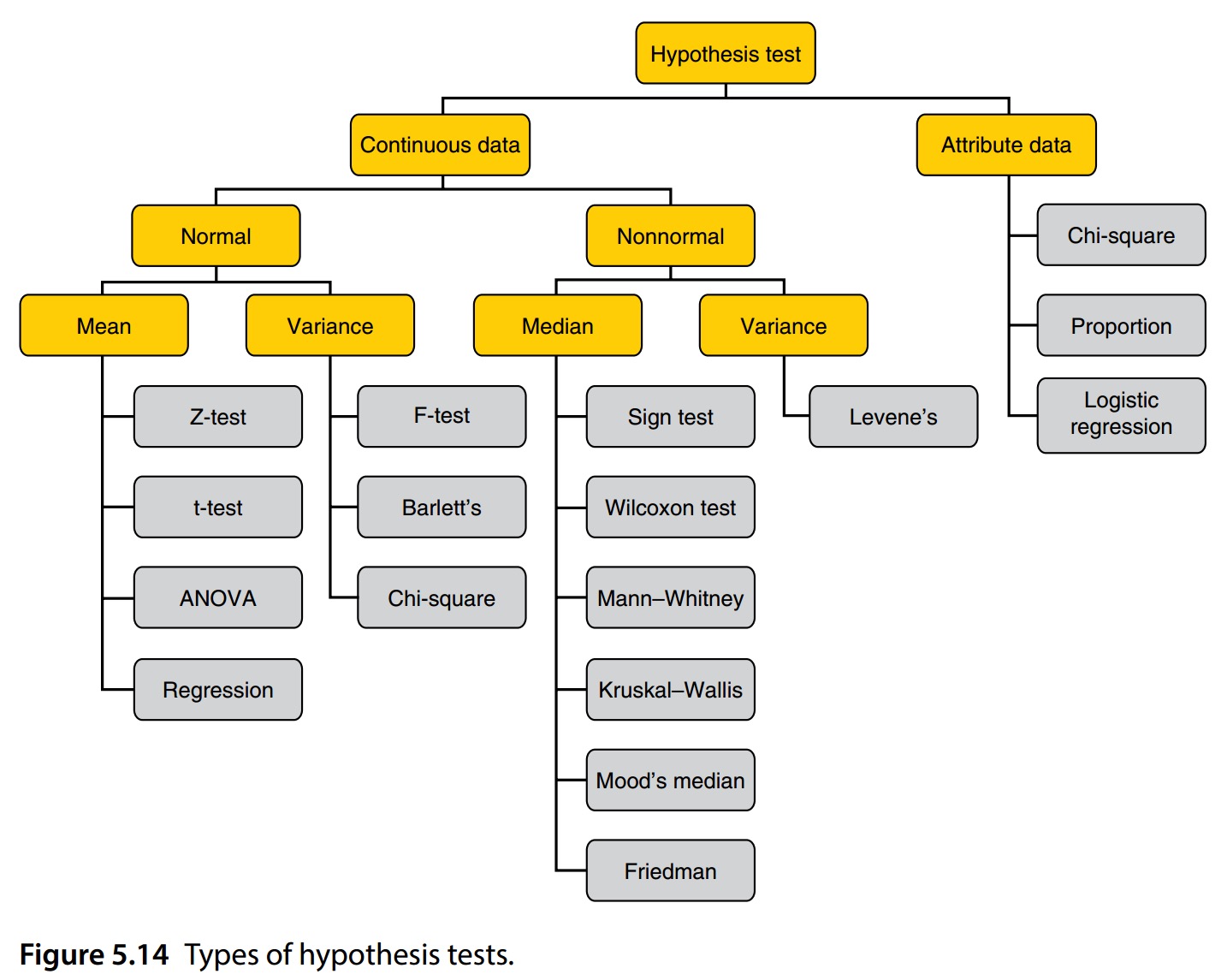
Test in Python Scipy stat
import scipy.stats as stats
oddsratio, pvalue = stats.fisher_exact([[8, 2], [1, 5]])
print oddsratio,pvalue
# 20.0 0.03496503496503495
test distribution
# test based on poisson distribution
def sig_test_poisson(x=0.3,mu=0.001):
p=stats.distributions.poisson.pmf(x,mu)
#print "poisson test:",p # work correctly
return p
# test based on negative binomial distribution
def sig_test_nbinom(x=100,n=50,p=0.3):
p=stats.distributions.nbinom.pmf(x,n,p)
#print "nbinom test:",p # work correctly
return p
KS test
# two sample distribution test
def ks_2samp(x,y):
p=stats.ks_2samp(x,y)[1]
return p
calculate correlation
# two sample rank test
def sig_spearman_corr(x,y):
p=stats.spearmanr(x,y)[0]
return p
def sig_pearson_corr(x,y):
p=stats.pearsonr(x,y)[0]
return p
rvs1 = stats.norm.rvs(loc=5,scale=10,size=500)
rvs2 = stats.norm.rvs(loc=5,scale=10,size=500)
stats.ttest_ind(rvs1,rvs2)
# (0.26833823296239279, 0.78849443369564776)
stats.ttest_ind(rvs1,rvs2, equal_var = False)
# (0.26833823296239279, 0.78849452749500748)
目录
bed file
Full description can be accessed at UCSC bed, here are example from bedtools introduction :
columns: 12 (some are optional correspond to different style)
- chrom - The name of the chromosome on which the genome feature exists.
- start - The 0-based starting position of the feature in the chromosome.
- end - The one-based ending position of the feature in the chromosome.
- name - Defines the name of the BED feature.
- score - The UCSC definition requires that a BED score range from 0 to 1000, inclusive.
- strand - Defines the strand - either ‘+’ or ‘-‘.
- thickStart - The starting position at which the feature is drawn thickly.
- thickEnd - The ending position at which the feature is drawn thickly.
- itemRgb - An RGB value of the form R,G,B (e.g. 255,0,0).
- blockCount - The number of blocks (exons) in the BED line.
- blockSizes - A comma-separated list of the block sizes.
- blockStarts - A comma-separated list of block starts.
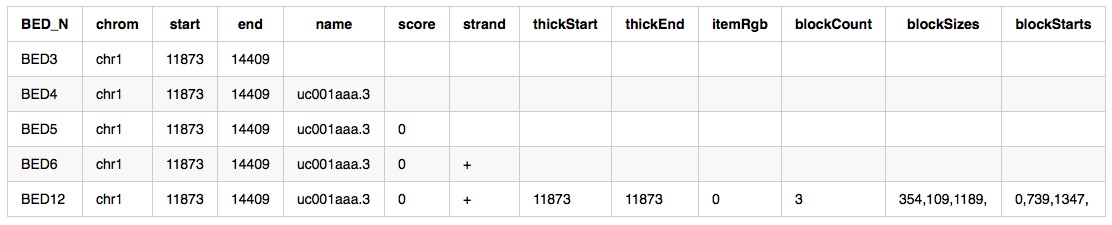
wig & bigwig file
- UCSC bigWig Track Format: https://genome.ucsc.edu/goldenpath/help/bigWig.html
- for dense, continuous data
- bigWig:
- indexed binary
- faster display performance
# convert .bw to .wig
bigWigToWig bigWigExample.bw out.wig
# large bedgraph to .bw
bedGraphToBigWig in.bedGraph chrom.sizes myBigWig.bw
Example:
$ head AGN001508.bedGraph AGN001508.wig
==> AGN001508.bedGraph <==
chr1 1720 1752 2.99808
chr1 6751 6760 2.99808
chr1 6891 6916 2.99808
chr1 13926 13969 2.99808
chr1 14504 14537 2.99808
chr1 14545 14555 2.99808
chr1 14555 14584 5.99616
chr1 14584 14586 2.99808
chr1 14586 14588 5.99616
chr1 14588 14596 2.99808
==> AGN001508.wig <==
#bedGraph section chr1:1720-25052994
chr1 1720 1752 2.99808
chr1 6751 6760 2.99808
chr1 6891 6916 2.99808
chr1 13926 13969 2.99808
chr1 14504 14537 2.99808
chr1 14545 14555 2.99808
chr1 14555 14584 5.99616
chr1 14584 14586 2.99808
chr1 14586 14588 5.99616
➜ seq_similarity head -3 outputfile_E10
ENST00000380087:1800-1900 ENST00000330735:600-700 100.00 66 0 0 166 35 100 1e-29 122
ENST00000380087:1800-1900 ENST00000330735:700-800 100.00 34 0 0 67100 1 34 6e-12 63.9
ENST00000557530:200-300 ENST00000348956:500-600 100.00 93 0 0 1 938 100 1e-44 172

some common used colors
seaborn
这里选取的颜色主要是来自于seaborn的,感觉这个颜色比较饱和,没有那么鲜艳:
category |
blue |
green |
red |
purple |
orange |
cyan |
red |
74 |
83 |
202 |
129 |
205 |
98 |
green |
113 |
169 |
75 |
112 |
185 |
180 |
blue |
178 |
102 |
78 |
182 |
111 |
208 |
HEX1 |
#4C72B0 |
#55A868 |
#C44E52 |
#8172B2 |
#CCB974 |
#64B5CD |
RGB |
74,113,178 |
83,169,102 |
202,75,78 |
129,112,182 |
205,185,111 |
98,180,208 |
指定获取不同颜色集合中的颜色列表,返回的列表可以用于后续的指定:
import seaborn as sns
def sns_color_ls():
return sns.color_palette("Set1", n_colors=8, desat=.5)*2
sns_color_ls = ['#4C72B0', '#55A868', '#C44E52', '#8172B2', '#CCB974', '#64B5CD']
参考:
python dict
source: Python Crash Course - Cheat Sheets
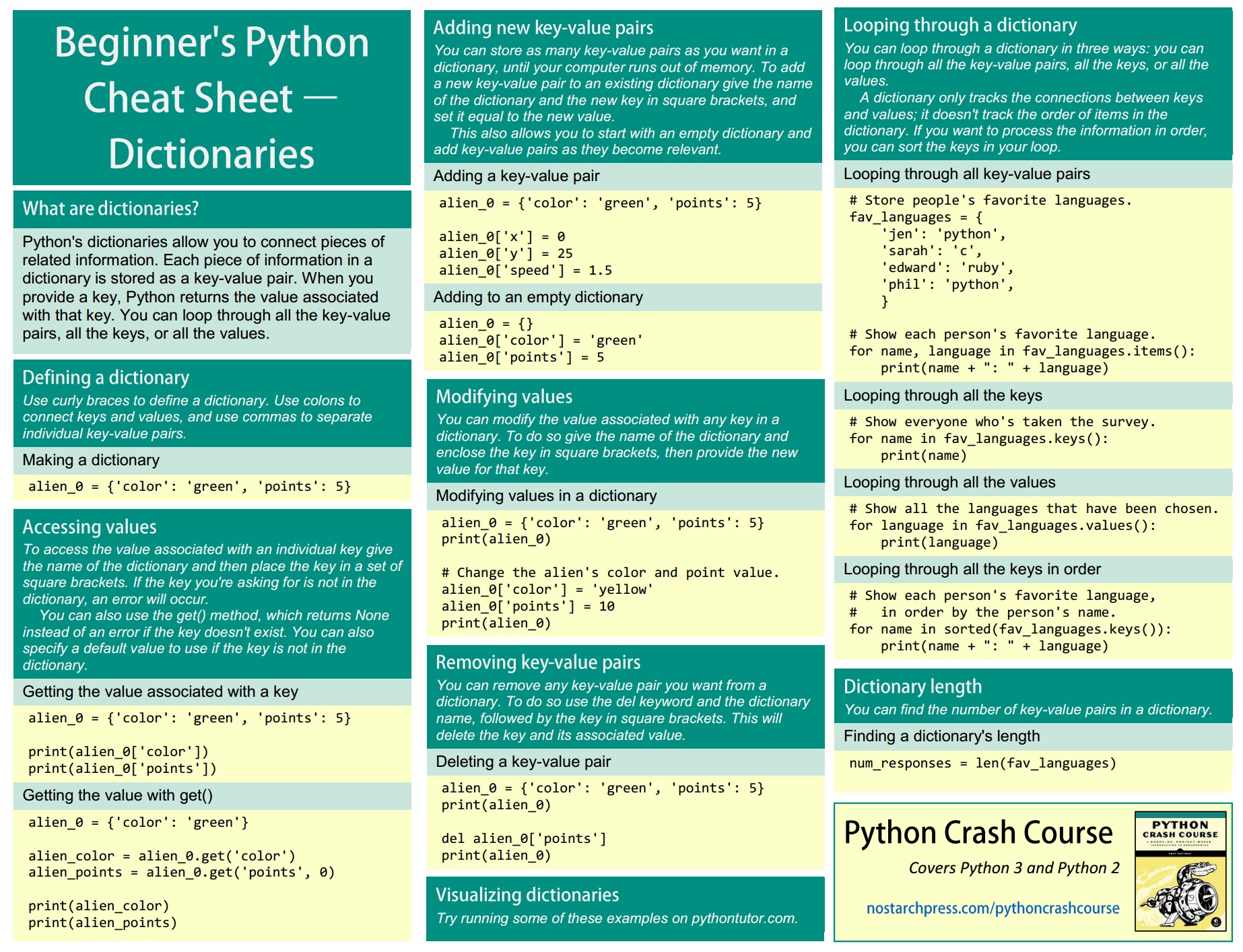
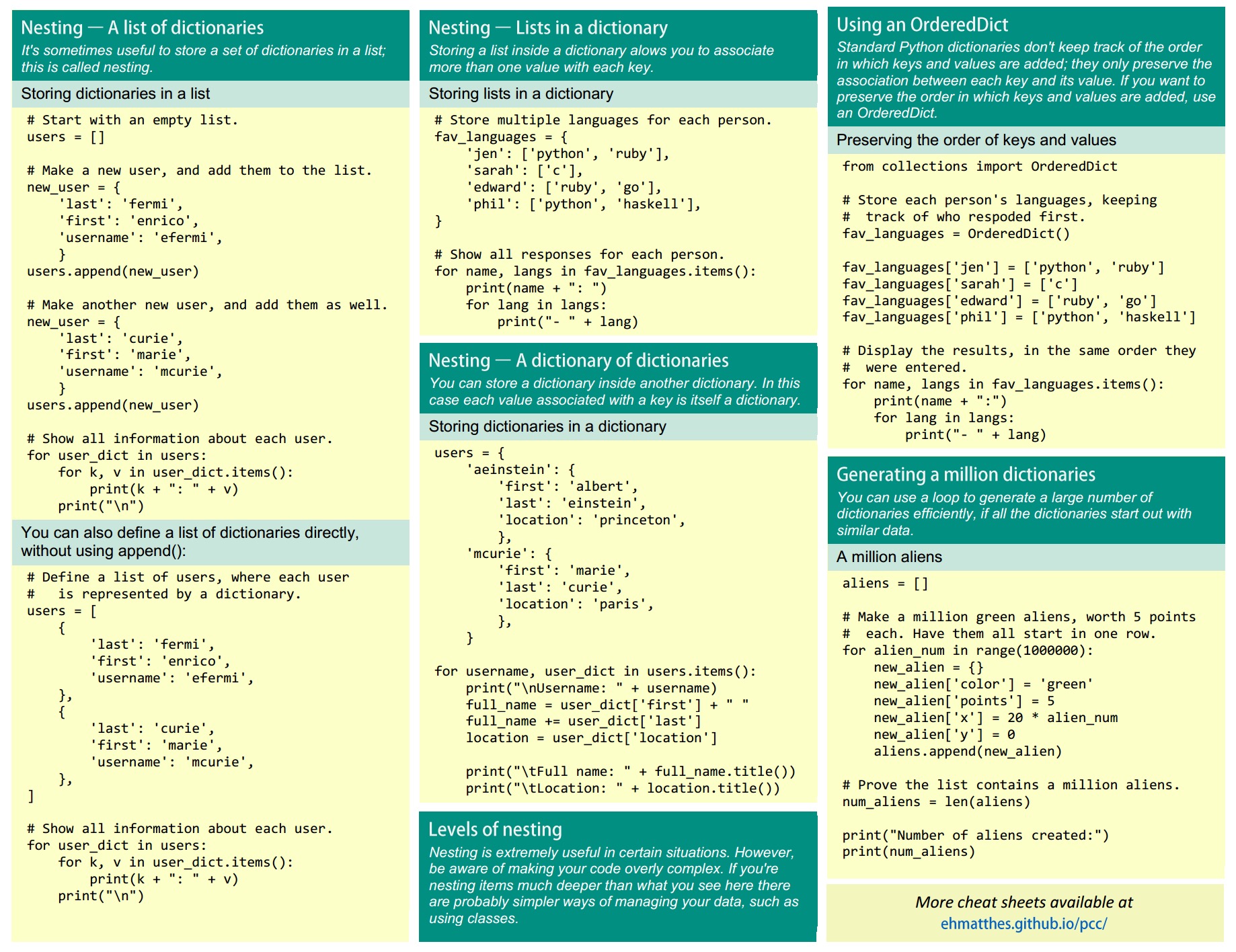
generate genome index for subsequent mapping
STAR index
runThreadN=12
genomeDir=/Share/home/zhangqf7/gongjing/zebrafish/data/reference/gtf/xiongtl/refseq_ensembl_homolog
genomeFastaFiles=/Share/home/zhangqf7/gongjing/zebrafish/data/reference/gtf/xiongtl/refseq_ensembl_homolog.fa
STAR --runThreadN $runThreadN \
--runMode genomeGenerate \
--genomeDir $genomeDir \
--genomeFastaFiles $genomeFastaFiles
output files:
[zhangqf7@bnode02 xiongtl]$ ll
total 1.1G
-rw-rw----+ 1 zhangqf7 zhangqf 8.3M May 18 15:31 Log.out
drwxrwx---+ 2 zhangqf7 zhangqf 4.0K May 18 15:31 refseq_ensembl_homolog
-rw-rw----+ 1 zhangqf7 zhangqf 139M May 18 15:23 refseq_ensembl_homolog.fa
-rwxrw----+ 1 zhangqf7 zhangqf 362 May 18 15:30 star_index.sh
drwx------+ 2 zhangqf7 zhangqf 4.0K May 18 15:31 _STARtmp
scp的用法
basic synatx:
scp [option parameter] file_source file_target
use the -p option to specific the port
scp -p port user@server_ip:/home/user/filename /home/user/filename
copy file from local to remote
file:
scp local_file remote_username@remote_ip:remote_folder
scp local_file remote_username@remote_ip:remote_file
scp local_file remote_ip:remote_folder
scp local_file remote_ip:remote_file
# first two command point the user and only password is
need later while the last two don't contains the user
information and the user name and password are both need
copy file from remote to local
scp root@www.cumt.edu.cn:/home/root/others/music /home/space/music/i.mp3
copy directory from local to remote
scp -r /home/space/music/ root@www.cumt.edu.cn:/home/root/others/
cp -r /home/space/music/ www.cumt.edu.cn:/home/root/others/
copy directory from remote to local
scp -r www.cumt.edu.cn:/home/root/others/ /home/space/music/
tricks
sort list in zip to keep relation order stackoverflow
>>> list1 = [3,2,4,1, 1]
>>> list2 = ['three', 'two', 'four', 'one', 'one2']
>>> list1, list2 = zip(*sorted(zip(list1, list2)))
>>> list1
(1, 1, 2, 3, 4)
>>> list2
('one', 'one2', 'two', 'three', 'four')
sort list of str or number
def sort_str_num_ls(ls=[1,2,3]):
if isinstance(ls[0],int):
return sorted(ls)
if isinstance(ls[0],str):
try:
return map(str,sorted([int(i) for i in ls]))
except:
return sorted(ls)
find index for a value
def find_all_value_index_in_list(lst=[1,2,3,4,5,1],f=1):
return [i for i, x in enumerate(lst) if x == f]
sum of list of list elements
def list_list_sum(lists=[[1,2],[3,4]],mode='count_sum'):
if mode == 'count_sum':
total=sum(sum(ls) for ls in lists)
if mode == "len_sum":
total=sum(len(ls) for ls in lists)
return total
flat nested list
def ls_ls_flat(ls_ls):
return list(itertools.chain.from_iterable(ls_ls))
convert value list to percentage list
# list to percent list
def list_pct(ls):
ls=map(float,ls)
ls_sum=sum(ls)
ls_pct=[i/ls_sum for i in ls]
return ls_pct
remove NA value of a list
def list_remove_na(ls):
return [i for i in ls if not np.isnan(i)]
shuffle a list with seed
参考这里:
>>> import random
>>> x = [1, 2, 3, 4, 5, 6]
>>> random.Random(4).shuffle(x)
>>> x
[4, 6, 5, 1, 3, 2]
>>> x = [1, 2, 3, 4, 5, 6]
>>> random.Random(4).shuffle(x)
>>> x
[4, 6, 5, 1, 3, 2]
sort a list based on anther list order
参考这里:
# ref: 需要参考的顺序list
# input:需要排序的list
[x for x in Ref if x in Input]
qucik cheatsheet
source: Python Crash Course - Cheat Sheets
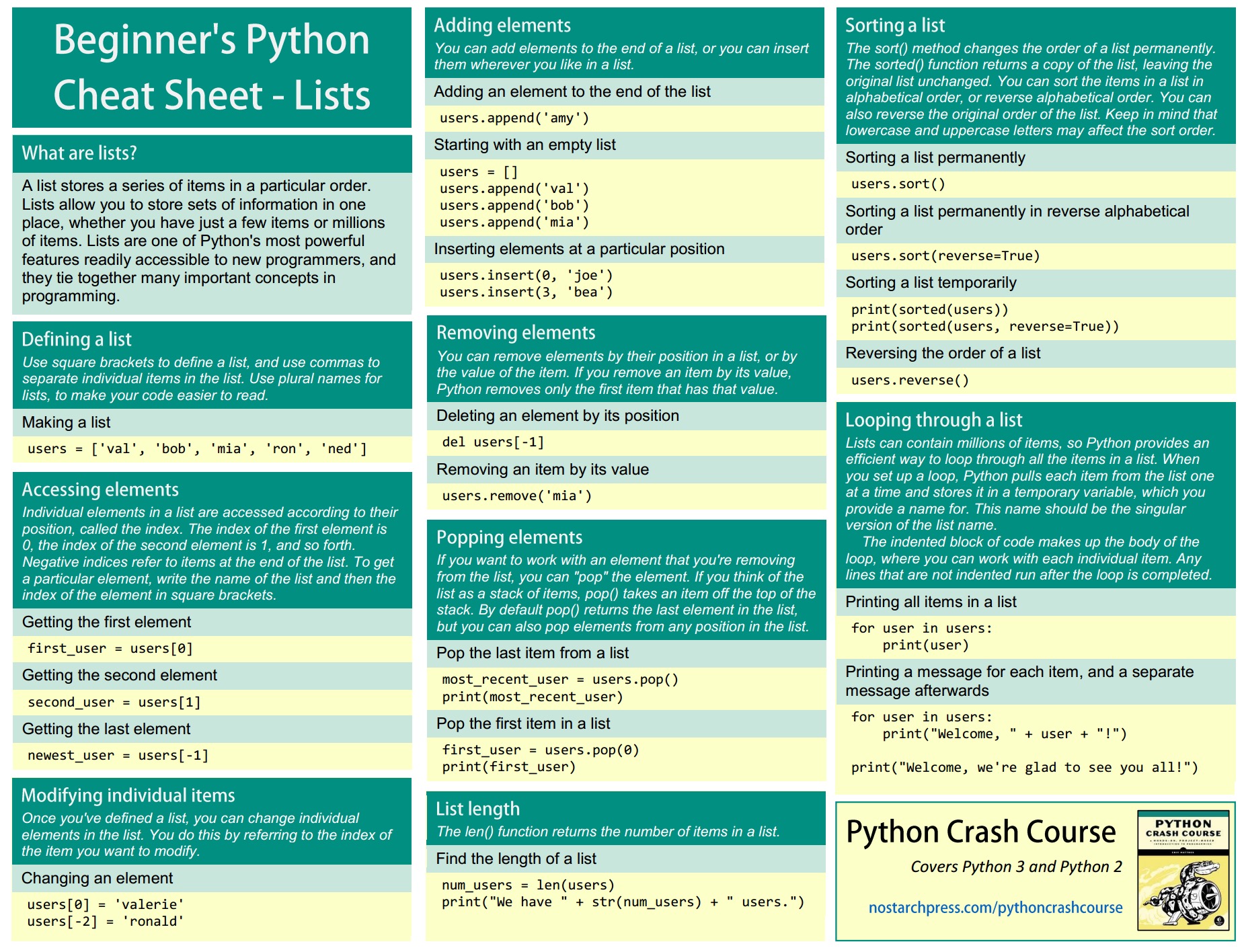
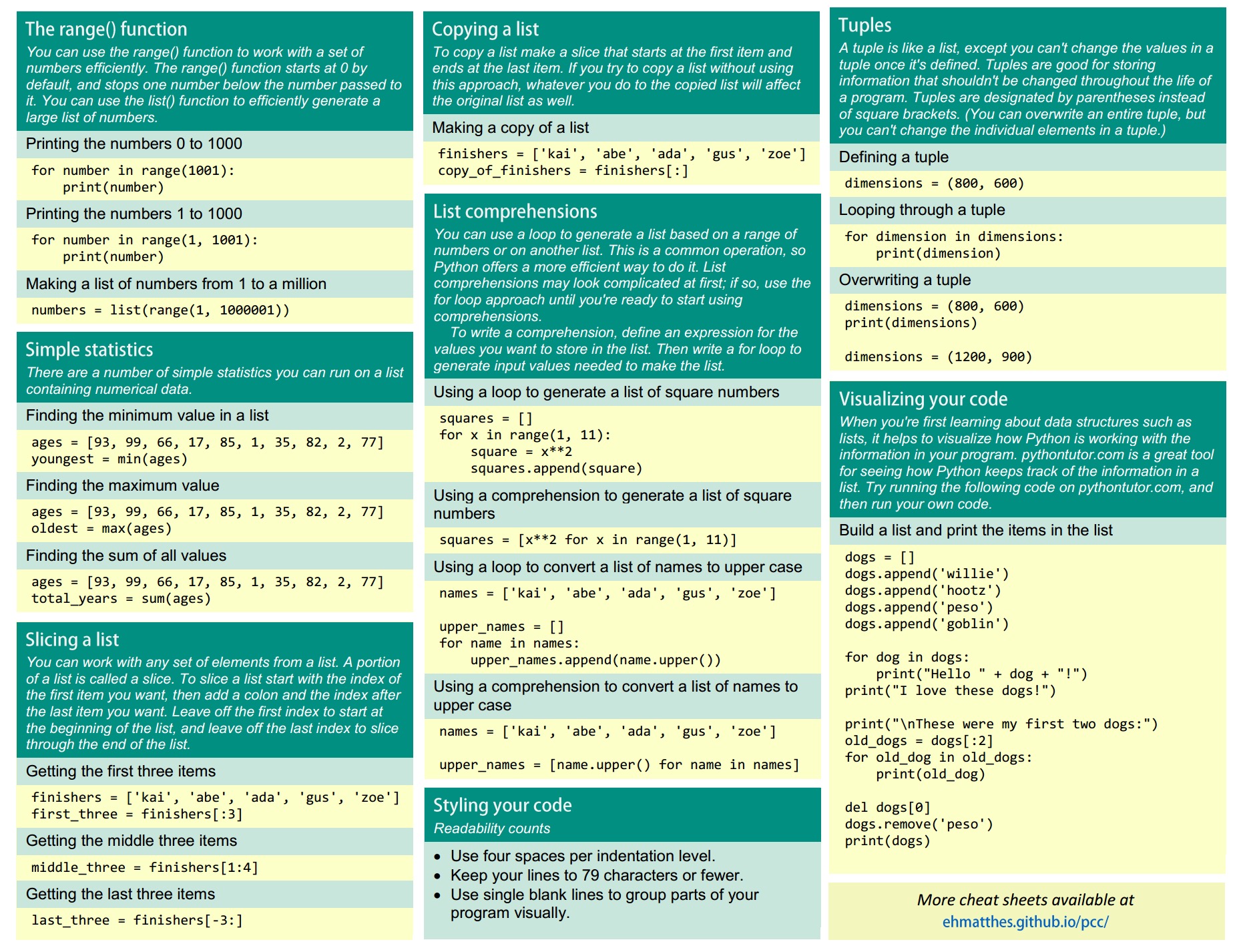
RNA biology
Mitchell Guttman, California Institute of Technology, labpage
- RAP-DNA, RAP-RNA
- The presence of thousands of functional large non-coding RNAs (lncRNAs) in the mammalian genome represents a missing component in our understanding of genome regulation.
- Our lab aims to understand how lncRNAs control gene expression programs and cell state decisions in the context of mouse embryonic stem cells. We are an integrated team of experimental and computational biologists who work together to address these questions using genomic approaches in conjunction with biochemistry, molecular biology, cell biology, and computational biology.
Xiangdong Fu, UCSD, labpage
- eCLIP, GRID-seq
- The Fu laboratory is interested in molecular and cell biology of RNA metabolism and regulation in higher eukaryotic cells. Current research interests in the Fu lab include the regulation of alternative splicing, functional RNA elements in mammalian genomes, transcription/splicing coupling, nuclear architecture, and cellular reprogramming.
Howard Chang, Stanford University, labpage
- lncRNA, ATAC-seq, icSHAPE, PARIS
- The Chang lab is focused on how the activities of hundreds or even thousands of genes (gene parties) are coordinated to achieve biological meaning. We have pioneered methods to predict, dissect, and control large-scale gene regulatory programs; these methods have provided insights into human development, cancer, and aging. A particular interest is how cells know and remember their locations in the body, particularly with the help of long noncoding RNAs.
RNA secondary structure
Alain Laederach, University of North Carolina, Chapel Hill, labpage
- RibosNitch, SNPfold
- The Laederach Lab is interested in better understanding the relationship between RNA structure and folding and human disease. We use a combination of computational and experimental approaches to study the process of RNA folding and in the cells. In particular, we develop novel approaches to analyze and interpret chemical and enzymatic mapping data on a genomic scale. We aim to fundamentally understand the role of RNA structure in controlling post-transcriptional regulatory mechanisms, and to interpret structure as a secondary layer of information (Nature comment, 2014). We are particularly interested in how human genetic variation affects RNA regulatory structure. We investigate the relationship between disease-associated Single Nucleotide Polymorphisms occurring in Human UTRs and their effect on RNA structure to determine if they form a RiboSNitch.
Kevin Weeks, University of North Carolina, Chapel Hill, labpage
- QuShape, Differential SHAPE, SHAPE-MaP, RING-MaP, deltaSHAPE, ShapeMapper 2
- Chemical Microscopes for High-Content RNA Structure Analysis
- Structure and Function in the Transcriptome
Anna Marie Pyle, Yale University, HHMI, labpage
- HOTAIR secondary structure, HCV secondary structure
- We explore RNA Biology, studying the molecular interactions important for RNA structures and the activities of RNA-protein machines. Using tools that range from crystallography to cell culture, we seek to understand the impact of RNA architecture and dynamics on the life of the cell.
Walter N. Moss, Iowa State University, labpage
- RNA2DMut, RNAStructuromeDB
- validate viral ncRNA’s predicted structures, identify interacting molecules, determine their localization within the cell and determine their effects on host cells.
- Viral cis-regulatory elements
- Host-virus interactions
Janusz M. Bujnicki, International Institute of Molecular and Cell Biology in Warsaw, Poland, labpage
- SimRNA: RNA 3D structure modeling (participate RNA Puzzles)
- Our group is involved in theoretical and experimental research on nucleic acids and proteins. The current focus is on RNA sequence-structure-function relationships (in particular 3D modeling), RNA-protein complexes, and enzymes acting on RNA.
Silvi Rouskin, MIT, labpage
- DMS-seq & DMS-MaPseq
- Separating alternative structures formed from the same underlying sequence (RNA Structure Control of Alternative Splicing)
Dan Herschlag, STANFORD UNIVERSITY, labpage
- RNA folding, RNA catalysis, protein catalysis, in vivo RNA structure and interactions
- We are particularly interested in questions of how enzymes work, how RNA folds, how proteins recognize RNA, and the roles of RNA/protein interactions in regulation and control, and the evolution of molecules and molecular interactions.
Sharon Aviran, UC Davis, labpage
- dStruct, patteRNA, RNAprob
- RNA structure and dynamics
- Our lab develops novel computational methods for inferring RNA dynamics from experiments and theory, with applications ranging from basic research to biomolecular engineering and synthetic biology.
David Mathews, University of Rochester, labpage
- RNAstructure
- Our goal is to automate the modeling of RNA structure and function from genome sequence to 3D structure.
Julius B. Lucks, Northwestern University, labpage
- SHAPE-Seq
- Pushing the Limits of RNA Design with Cellular Engineering
- Next Generation RNA Structure Characterization
- Harvesting RNA Design Principles from Nature: RNA-protein interaction
Danny Incarnato, University of Groningen (The Netherlands), labpage
- RNA folding dynamics research
- CIRS-seq, RNAframework
Salvatore Oliviero, Italian institute fro genomic medicine, labpage
- CIRS-seq
- Main aim of our lab is the understanding of the mechanisms controlling the different histone modifications, and the deciphering of the histone code which contributes to the transcriptional control, to understand and eventually influence cells destiny.
RNA-RNA interaction
Zhipeng Lu, University of Southern California School of Pharmacy, labpage
- PARIS
- Our research is focused on “RNA machines” in living cells. We develop and apply novel technologies to understand the structures and functions of RNA molecules in basic cellular processes, with the ultimate goal of treating human diseases, including genetic disorders, cancers and viral infections.
Irmtraud Margret Meyer, Max-Delbrück-Centrum für Molekulare Medizin, labpage
RNA-protein interaction
Gene Yeo, UCSD, labpage
- eCLIP, CLIPPER
- how RNA binding proteins and RNA modifications affect cellular homeostasis in human pluripotent stem cells.
- how defects in RNA binding proteins cause neurological disease, such as ALS.
- post-transcriptional processing of RNAs by multiple mechanisms.
- develop new computational approaches to decipher biological meaning from thousands of single-cell RNA-seq/proteomics data.
RNA-DNA interaction
Sheng Zhong, UCSD, labpage
- We study gene regulation and cellular behavior by developing statistical and experimental methods. Our primary goal is to develop new technologies to map molecular networks, including RNA-RNA interactome [MARIO, Nat Comm, 2016], RNA-chromatin interactome [MARGI, Curr Biol, 2017], and protein-protein interactome. Our secondary quest is to model the variations of these networks in three axes, namely developmental time, personal difference, and evolutionary change. Our major tools include epigenomic and single-cell assays, single-molecule imaging, statistical modeling, and large scale computation.
Stress granule
Roy Parker, UNIVERSITY OF COLORADO BOULDER, labpage
- Our goal is to understand the molecular mechanisms that control mRNA stability and translation rate in eukaryotic cells, using yeast as a model system.
Jernej Ule, UCL/Crick institute, labpage
- HiCLIP, iCLIP, RNP granule
- The goal of our research group is to reveal how RNPs regulate the life cycle of mRNAs in neurons, and how this can go wrong in neurologic diseases. To study the assembly of RNPs, we obtain detailed maps of protein-RNA binding sites by using transcriptomic techniques. For this purpose, we developed the nucleotide-resolution UV crosslinking and immunoprecipitation (iCLIP), which identifies protein-RNA contacts by using a series of steps, as described in the figure above. We are further developing similar methods, as well as computational tools to interpret the high-throughput sequencing data. Thereby, we gain a comprehensive view of RNP assembly and dynamics within intact cells.